Last update : June 25, 2021
Coqui.ai and Rhasspy are two outstanding projects, dealing with modern speech technologies and sharing their open-source code on GitHub. Coqui.ai states that it frees speech technology and brings research into reality. Rhasspy provides a fully offline set of voice assistant services for home automation.
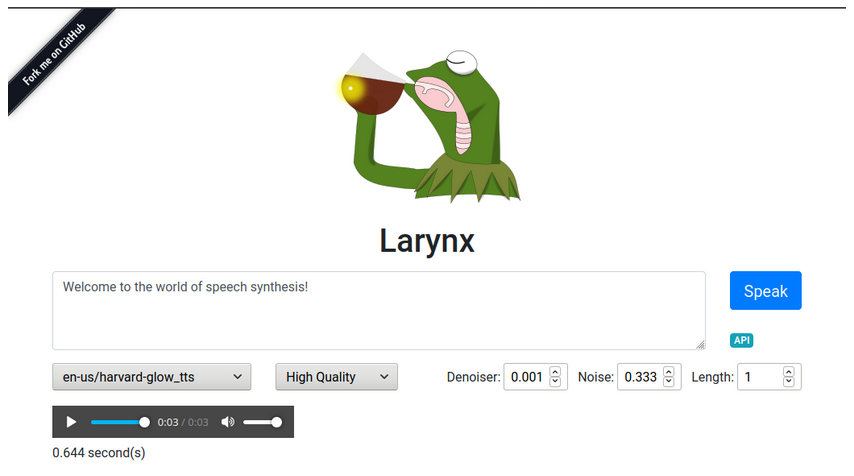
Both projects have released TTS (Text-to-Speech) and STT (Speech-to-Text) models for several languages, based on deep machine learning. Rhasspy has developed an excellent system for grapheme to phoneme conversion (sort of frontend) called gruut and offers a flexible output interface (sort of backend), with various intent recognition systems and intent handling systems. The focus of Coqui.ai is the research, development and teaching of different ML (Machine Learning) models for TTS and STT : Tacotron-DCA, Tacotron2-DDC, Glow-TTS, SpeedySpeech, DeepSpeech etc.
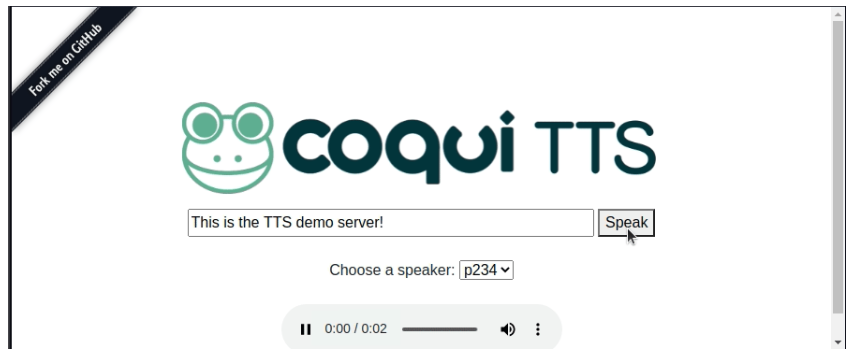
Recently, the main developer of Coqui-TTS, Eren Gölge, alias erogol, and the creator of Rhasspy, Michael Hansen, alias synesthesiam, worked together to integrate gruut into Coqui-TTS. The GitHub pull request, prepared by synesthesiam, was ready for merging on June 17, 2021. I am very interested to work with this combined TTS release to eventually create a synthetic voice for the luxembourgish language.
A few days ago, I cloned the branch add-gruut of the Coqui-TTS GitHub repository, forked by synesthesiam, on my Desktop-PC to check if the new tool allows to train a synthetic voice, with a small dataset, during a limited training period.
I used a part (4.500 utterances) of the french public SIWIS dataset to train a Glow-TTS model. My hyper-parameters to configure the model are based on the Rhasspy-Larynx configuration file of the french Larynx Glow-TTS model, trained with the full SIWIS dataset.
My configuration file can be downloaded from this link.
The model was trained with 1000 epochs with a batch size of 45, which equals to 99.000 steps. The main scalars and images displayed on the Tensorboard at the end of the training are shown hereafter :
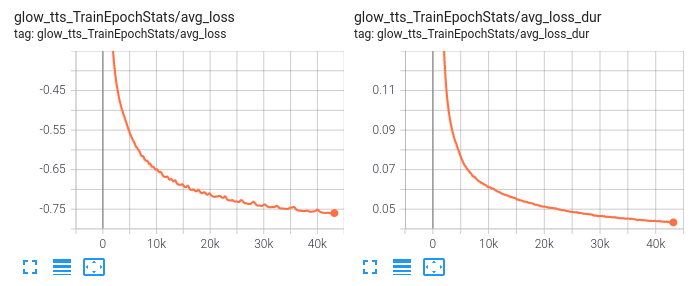
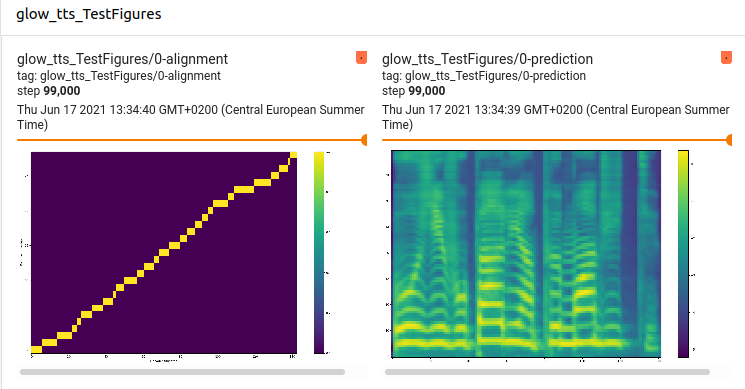
The final model is available here : fr-siwis-coqui-glow-99k.pth.tar
I run the following script to synthesize the french version of the fable The North Wind and the Sun :
tts --text "La bise et le soleil se disputaient, chacun assurant qu'il était le plus fort, quand ils ont vu un voyageur qui s'avançait, enveloppé dans son manteau. Ils sont tombés d'accord que celui qui arriverait le premier à faire ôter son manteau au voyageur serait regardé comme le plus fort. Alors, la bise s'est mise à souffler de toute sa force mais plus elle soufflait, plus le voyageur serrait son manteau autour de lui et à la fin, la bise a renoncé à le lui faire ôter. Alors le soleil a commencé à briller et au bout d'un moment, le voyageur, réchauffé, a ôté son manteau. Ainsi, la bise a dû reconnaître que le soleil était le plus fort des deux." \
--model_path /home/mbarnig/myTTS-Project/experiments/fr-siwis-part1-glow-99k.pth.tar \
--config_path /home/mbarnig/myTTS-Project/experiments/fr-siwis-part1-glow-config.json \
--out_path /home/mbarnig/myTTS-Project/experiments/bise-et-soleil-gl.wav
Here are the results when using the default Griffin-Lim vocoder :
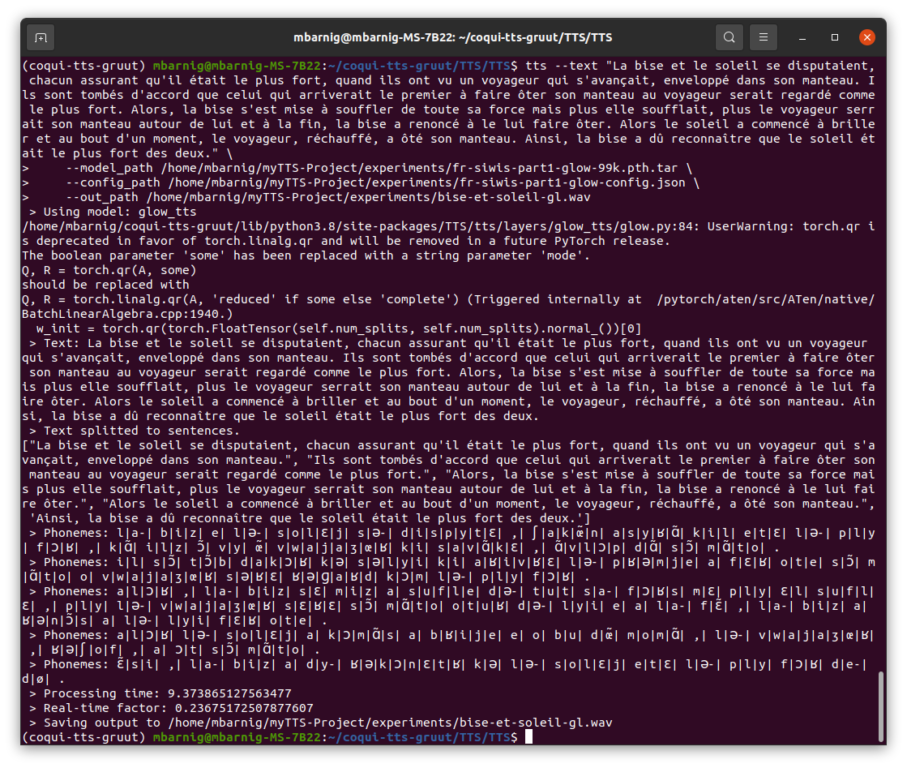
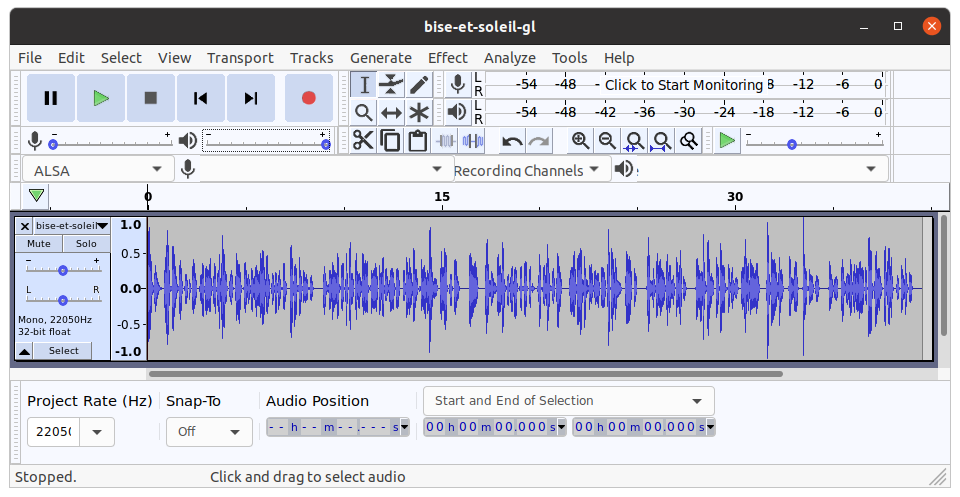
To improve the voice we must continue the training. The SIWIS Larynx voice, released by Rhasspy, was trained with 270.000 steps and more utterances.
Below the audio signals and sounds of the SIWIS voice, trained with 195.000 steps, are displayed:
