Mise à jour : 15 avril 2022
Introduction
En 1960, J.C.R. Licklider a publié son fameux article au sujet de la symbiose homme-machine qui préfigurait l’informatique interactive. Huit ans plus tard, en 1968, il publiait, ensemble avec Robert W. Taylor, la contribution visionnaire The Computer as a Communication Device. Le dessin humoristique qui suit, réalisé à l’époque pour cette publication par Rowland B. Wilson, montre l’ordinateur OLIVER qui annonce à un visiteur que son patron n’est pas au bureau.
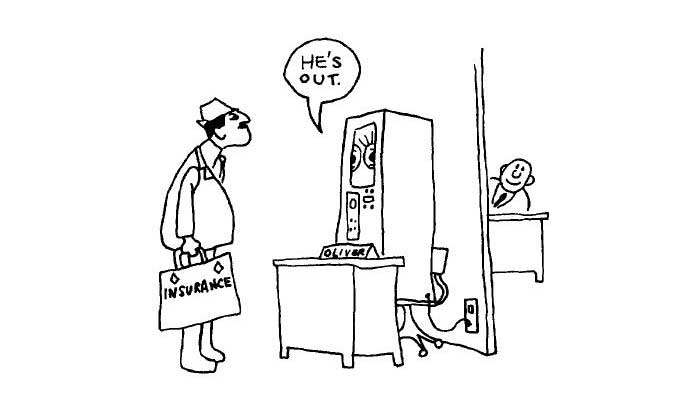
“The Computer as a Communication Device”
Toutefois il fallait attendre presque 40 ans avant que cette vision soit devenue une réalité. Aujourd’hui la reconnaissance de la parole humaine et la synthèse vocale sont des tâches quotidiennes effectuées par les ordinateurs. La parole devient de plus en plus importante pour la communication Homme-Machine.
Hélas la majorité des humains est encore écartée de la facilité de s’entretenir avec un ordinateur dans sa langue maternelle. Des interfaces vocales performantes sont disponibles depuis quelques années pour les langues les plus parlées : anglais, mandarin, hindi, espagnol, français, allemand, … Plus que la moitié de la population mondiale parle une des autres 7.000 langues qui existent sur la planète. Parmi ce groupe il y a de nombreuses langues à faibles ressources qui ne disposent pas de règles pour l’écriture ou pour la vocalisation.
Bien que le Luxembourgeois a obtenu en 1984 le statut de langue nationale, dispose d’une orthographie, grammaire et phonologie standardisées, d’un commissaire à la langue luxembourgeoise, d’un Conseil permanent de la langue luxembourgeoise, du Zenter fir d’Lëtzebuerger Sprooch, d’un Institut National des Langues, d’une chaire de luxembourgeois à l’Université du Luxembourg, d’un outil informatique Spellchecker et d’un outil de traduction Google, il n’est pas supporté par les interfaces vocales (Alexa, Siri, Cortona, … ) proposées par les géants du web (GAFAM’s) ou par d’autres fournisseurs de systèmes informatiques.
Marylux
Depuis l’antiquité les humains ont été fascinés par les technologies de synthèse vocale. J’ai raconté l’histoire de ces technologies dans mon livre Synthèse vocale mécanique, électrique, électronique et informatique. Un des systèmes TTS (Text-to-Speech) informatiques les plus universels et performants est MaryTTS de l’université de la Sarre, dont le développement remonte à l’année 2000. En 2015, la langue luxembourgeoise a été ajoutée à ce système sous le nom de Marylux. La base de données Marylux comporte un fichier audio enregistré en 2014 par Judith Manzoni sur base de phrases luxembourgeoises (63 minutes), françaises (47 minutes) et allemandes (22 minutes), avec les textes correspondants.
Pour donner une idée sur la qualité de la voix synthétique Marylux je vais présenter l’enregistrement original et la génération synthétique par MaryTTS de la fable d’Esope “Borée et le Soleil” en langue luxembourgeoise. Voici le texte de cette fable :
Den Nordwand an d’Sonn.An der Zäit hunn sech den Nordwand an d’Sonn gestridden, wie vun hinnen zwee wuel méi staark wier, wéi e Wanderer, deen an ee waarme Mantel agepak war, iwwert de Wee koum. Si goufen sech eens, datt deejéinege fir dee Stäerkste gëlle sollt, deen de Wanderer forcéiere géif, säi Mantel auszedoen. Den Nordwand huet mat aller Force geblosen, awer wat e méi geblosen huet, wat de Wanderer sech méi a säi Mantel agewéckelt huet. Um Enn huet den Nordwand säi Kampf opginn. Dunn huet d’Sonn d’Loft mat hire frëndleche Strale gewiermt, a schonn no kuerzer Zäit huet de Wanderer säi Mantel ausgedoen. Do huet den Nordwand missen zouginn, datt d’Sonn vun hinnen zwee dee Stäerkste wier.
On peut écouter ci-après la parole enregistrée
et la parole synthétisée de ce texte avec MaryTTS :
avec la base de données Marylux
Les figures suivantes montrent l’évolution du son dans le temps pour la première phrase de la fable, ainsi que les spectrogrammes correspondants :
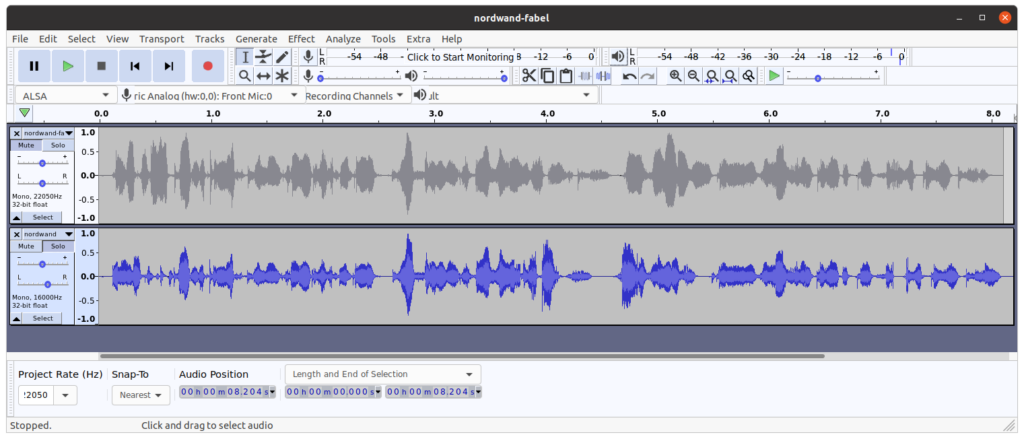
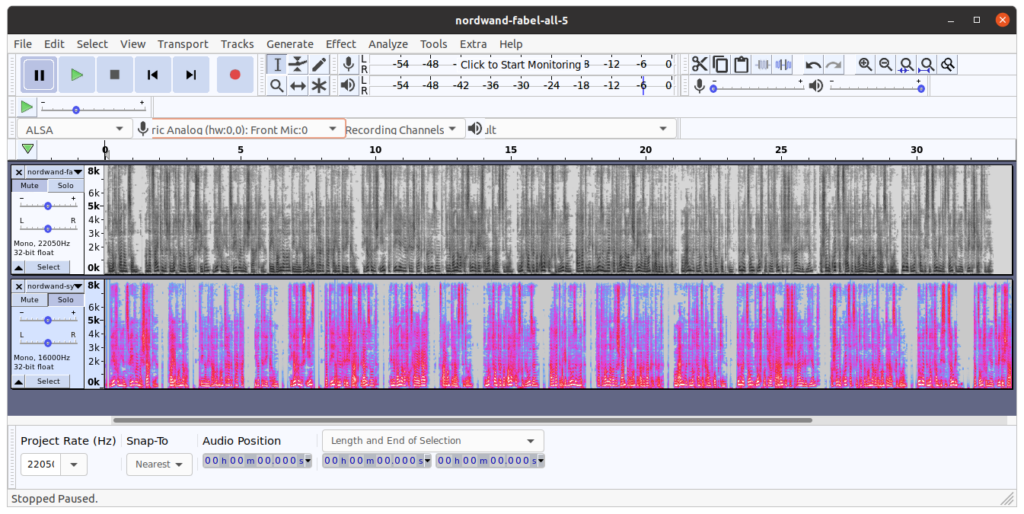
Synthèse vocale avec réseaux neuronaux
La technologie de synthèse Marylux reposait sur un modèle informatique utilisant une méthode de sélection d’unités. Cette technique est devenue obsolète aujourd’hui, suite à la progression fulgurante des technologies d’apprentissage approfondi des machines (deep machine learning) sur base de réseaux neuronaux.
Lors de la conférence Interspeech 2017 à Stockholm, Google a présenté un nouveau système de synthèse vocale appelé Tacotron qui repose sur la superposition de deux réseaux neuronaux. Les résultats étaient proches d’une prononciation par des humains. La publication académique afférente porte les noms de 14 auteurs.
Depuis cette date ce modèle a été perfectionné et de nouveaux modèles neuronaux TTS ont été développés : Tacotron2-DCA, Tacotron2-DDC, GlowTTS, Fast-Pitch, Fast-Speech, AlignTTS, Speedy-Speech, VITS, … En 2021 on a pu découvrir tous les quelques jours une nouvelle publication scientifique au sujet de TTS dans l’archive ouverte de prépublications électroniques ArXiv sur Internet.
Marylux-648
Pour entraìner un modèle TTS neuronal avec la base de données Marylux, enregistrée en 2014, j’ai ajusté les fichiers correspondants pour les adopter d’une façon optimale aux besoins de l’apprentissage automatique profond (deep machine learning). J’ai publié la nouvelle base de données publique sous le nom de Marylux-648-TTS-Corpus sur mon dépôt de développement Github. Il s’agit de 648 clips audio luxembourgeois, chacun ayant une durée inférieure à 10 secondes, et des transcriptions y associées. La durée totale est de 57 minutes et 31 secondes. Le lecteur intéressé est invité à consulter les détails sur Github.
Comme les modèles TTS neuronaux sont gourmands en données et entraînés en général avec des enregistrements audio d’une durée de plusieurs dizaines d’heures, j’ai exploré dans une première phase le potentiel et les contraintes de différents modèles TTS face à une base de données dont la durée totale n’atteint même pas une heure.
Avant de présenter les résultats de mes premiers tests, je vais décrire sommairement le cadre de mes expériences.
Modèles TTS neuronaux à source ouverte
Les modèles TTS neuronaux les plus courants peuvent être répartis en quatre grandes familles:
- Tacotron
- Glow
- Forward-Feed
- Vits
Pour les trois premières familles, la synthèse (inference) de la parole à partir d’un texte se fait en deux étapes. Le texte est d’abord converti en spectrogramme, puis transformé en signal audio avec un vocodeur (vocoder). Les vocodeurs les plus courants sont relevés ci-après:
- Griffin-Lim
- WaveNet
- WaveRNN
- WaveGrad
- WaveGAN
- HifiGAN
- MelGAN
- UnivNet
À l’exception du premier vocodeur (Griffin-Lim) qui est algorithmique, les autres vocodeurs sont également entraìnés avec des réseaux neuronaux sur base d’enregistrements audio, de préférence avec la même base de données que celle utilisée pour l’apprentissage du modèle TTS. Hélas les différents vocodeurs ne sont pas tous compatibles avec les différents modèles TTS.
La quatrième famille (VITS) dispose d’une architecture de bout-à-bout (end-to-end) avec vocodeur intégré.
A côté du type de modèle TTS et du type de vocodeur, on fait la distinction entre les caractéristiques suivantes:
- support d’une seule langue avec une voix (mono-speaker)
- support d’une seule langue avec plusieurs voix (multi-speaker)
- support de plusieurs langues avec une voix (multilingual, mono-speaker)
- support de plusieurs langues avec plusieurs voix (multilingual, multi-speaker)
En ce qui concerne le choix des modèles TTS et des vocodeurs pour mener mes tests, je me suis limité à l’utilisation de projets tiers à source-ouverte (open-source) et avec des licences libres de droits (MIT, CC, …). À côté de projets de développeurs individuels que je vais présenter dans la suite, je me suis notamment basé sur les développements réalisés par les communautés Coqui-TTS et Rhasspy.
Entraînement d’un modèle TTS avec une nouvelle base de données
L’entraînement d’un modèle TTS avec une nouvelle base de données peut se faire à partir de zéro (from scratch) ou à partir d’un modèle existant. Dans le deuxième cas on parle de transfert d’apprentissage (transfer learning) ou de fin réglage (fine tuning).
Les 648 échantillons de la base de données Marylux-648 sont d’abord mélangés (shuffling), puis répartis en 640 exemples pour l’entraînement proprement dit et en 8 exemples pour l’évaluation, effectuée après chaque cycle d’apprentissage. Les six phrases de la fable “De Nordwand an d’Sonn”, qui ne font pas partie du jeu d’apprentissage, sont utilisées pour les tests automatiques réalisés après chaque évaluation.
Pour l’entraînement des modèles j’utilise les infrastructures suivantes:
- mon ordinateur personnel avec carte graphique NVIDIA RTX 2070, système d’exploitation Linux Ubuntu 20.4 et système de développement Python 3.8
- mon compte Google-Colab pro dans les nuages, avec CUDA P100 et système de développement Python 3.7
Un cycle d’apprentissage complet est appelé une époque (epoch). Les itérations sont effectuées par lot (batch). La durée d’une itération est fonction de la taille du lot. On a donc intérêt à choisir une taille élevée pour un lot. La différence s’exprime par des durées d’apprentissage de plusieurs heures, jours, semaines ou voire des mois. Hélas la taille des lots est tributaire de la taille de mémoire disponible sur la carte graphique (CUDA).
En général je ne peux pas dépasser une taille de lot supérieure à 10 sur mon ordinateur personnel, sans provoquer une interruption de l’entraînement à cause d’un débordement de la mémoire (memory overflow). Sur Google-Colab je ne peux guère dépasser une valeur de 32.
Pour faciliter la comparaison des performances et qualités des différents modèles TTS, j’ai défini une limite de 1000 époques pour chaque entraînement. Sur mon ordinateur personnel une époque prend donc 64 itérations, sur Google-Colab le nombre se réduit à 20. Le temps d’exécution d’une époque est en moyenne de 95 secondes sur mon ordinateur personnel, ce qui fait environ 26 heures pour l’entraînement complet d’un modèle TTS avec Marylux-648 (64.000 itérations).
Avec un lot de 32, on s’attend à une réduction du temps d’entraînement d’un facteur 3,2, c.à.d. à environ 8 heures. Or à cause du partage des ressources entre plusieurs utilisateurs sur Google-Colab, le gain est plus faible. J’ai observé un temps de calcul moyen de 72 secondes par époque, ce qui donne une durée totale d’entraînement d’environ 20 heures pour 1000 époques (20.000 itérations).
Pour entraîner une base de données de référence comme LJSpeech, VCTK ou Thorsten Voice, qui ont chacune une durée d’enregistrement d’environ 24 heures, le temps de calcul se situe donc entre 3 et 4 semaines, dans les mêmes conditions. On peut réduire le temps d’apprentissage en augmentant la taille des lots à 64, voire à 128 échantillons. Une autre possibilité consiste à utiliser plusieurs cartes graphiques connectées en réseau. Le projet Coqui-TTS supporte une telle interconnexion CUDA. Des témoignages recueillis auprès de développeurs de modèles TTS neuronaux confirment que dans la pratique il faut compter une à deux semaines pour exécuter un entraînement avec une nouvelle base de données, ayant une durée d’enregistrements d’une dizaine d’heures, sur un ensemble ordinateur & CUDA performant.
Marylux-648 Tacotron2-DCA
Pour présenter les résultats obtenus avec l’entraînement de la base de données Marylux-648, il convient de commencer avec le doyen des modèles TTS neuronaux: Tacotron. L’apprentissage profond a été réalisé à partir de zéro. Avant d’entrer dans les détails, nous allons écouter la synthèse de la fable “De Nordwand an d’Sonn”.
Le résultat n’est pas fameux !
La prochaine image montre la séquence temporelle des signaux audio. La figure confirme qu’il y a problème.
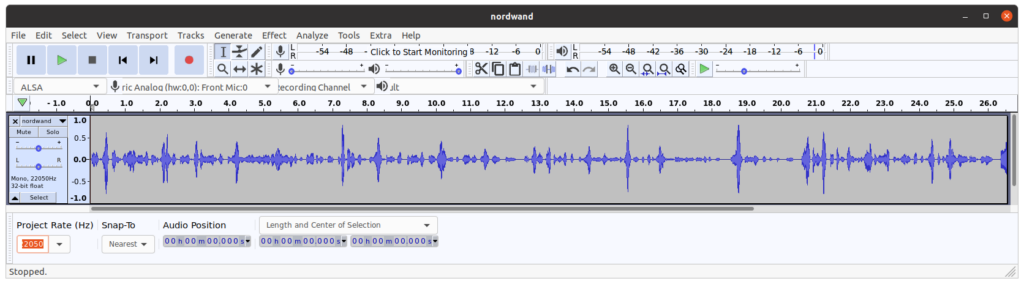
En utilisant un vocodeur entraìné au lieu du vocodeur algorithmique Griffin-Lim, on peut améliorer la qualité de la synthèse. La première phrase de la fable Nordwand sert d’échantillon de comparaison. La synthèse avec vocodeur Griffin-Lim est reprise ci-après, suivie par la synthèse avec un vocodeur Hifigan.
Le signal temporel de ces deux clips audio est affiché dans l’image qui suit. Une différence notable entre les deux clips est bien visible, mais la qualité auditive n’est pas encore satisfaisante.
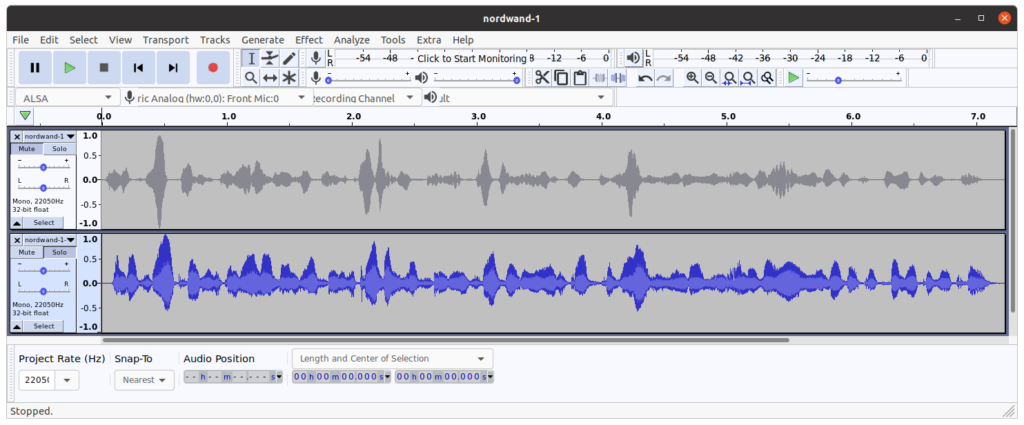
Le modèle Coqui-TTS Tacotron2-DCA (Dynamic Convolution Attention) constitue une n-ième évolution du premier modèle TTS Tacotron avec une nouvelle architecture qui est cofigurée par un ensemble de plus de 150 hyper-paramètres, en format json. La liste complète de configuration peut être téléchargée sur mon site web, un extrait est présenté dans la figure qui suit.
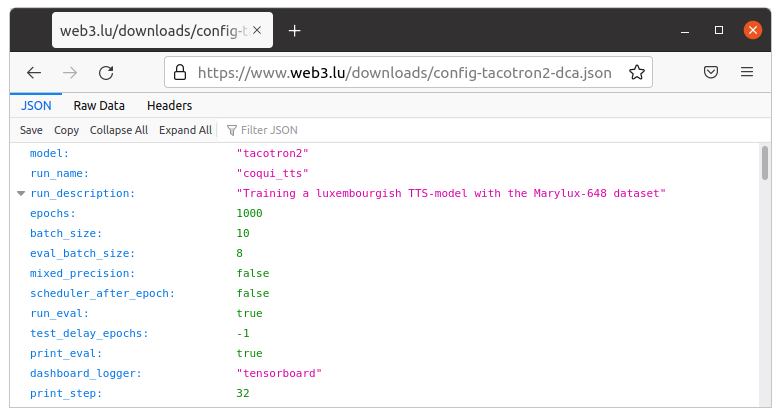
Une possibilité théorique d’améliorer la qualité de synthèse d’un modèle TTS est de mieux régler la configuration, suivant une procédure “essai-erreur (trial and error)” ou sur base d’analyses complexes supplémentaires de la base de données. Avec le grand nombre de paramètres de configuration et les durées d’entraînement dépassant une journée pour effectuer un nouveau essai avec la base de données Marylux-648, ce n’est guère praticable.
Une deuxième possibilité consiste à prolonger l’apprentissage en continuant l’entraînement pendant plusieurs époques supplémentaires. Mais comme dans notre cas le dernier fichier de contrôle (checkpoint) avec le nom “best_model.pth.tar” a été enregistré après xxx itérations (steps), cela ne fait pas de sens de dépasser mon seuil fixé à 1000 époques (64.000 itérations).
La seule solution valable consiste à étudier en détail la progression du livre de bord (logs), enregistré lors de l’entraînement. Le modèle TTS Tacotron2-DCA de coqui.ai, comme d’ailleurs tous les modèles TTS partagés par cette jeune start-up, utilisent le fameux kit de visualisation de TensorFlow, appelé TensorBoard. L’outil permet le suivi et la visualisation de métriques relatifs à l’apprentissage profond (deep machine learning), telles que la perte (loss) et la justesse. TensorBoard peut également afficher des histogrammes de pondérations et de biais, des images, des textes et des données audio, au fur et à mesure de leur évolution.
Les métriques générés lors de l’entraînement du modèle TTS Tacotron2-DCA permettent l’affichage, sous forme graphique, de la progression des résultats relatives aux évaluations (13 scalaires, 4 images, 1 fichier audio), aux époques, respectivement aux tests (16 scalaires, 12 images, 6 fichiers audio) et aux itérations, respectivement à l’entraînement (16 scalaires, 4 images, 1 fichier audio).
Les trois images qui suivent donnent un aperçu sur l’affichage de scalaires, d’images (spectrogrammes et attentions) et de fichiers audio du modèle TTS Tacotron2-DCA, à la fin de l’entraînement avec la base de données Marylux-648.
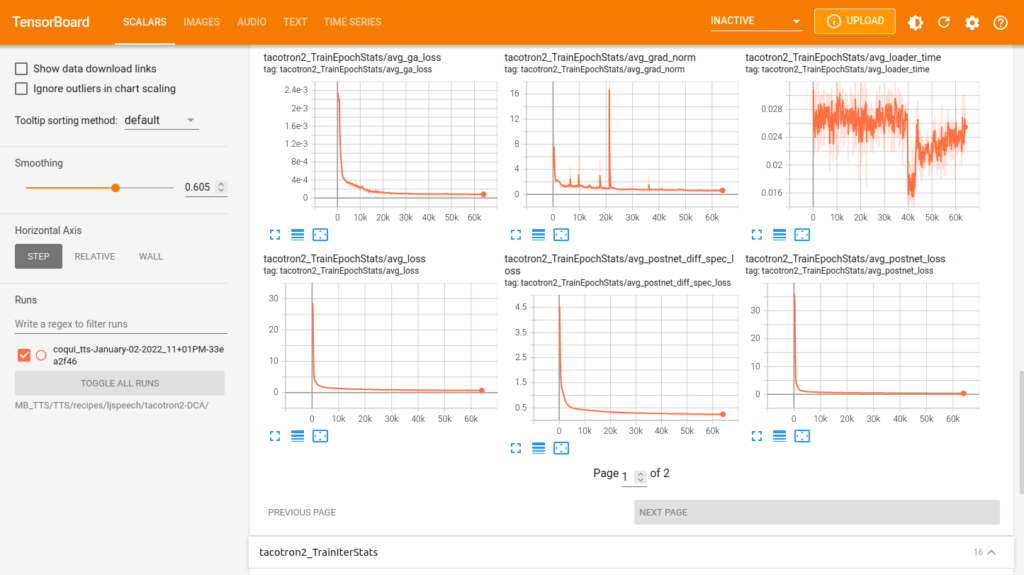
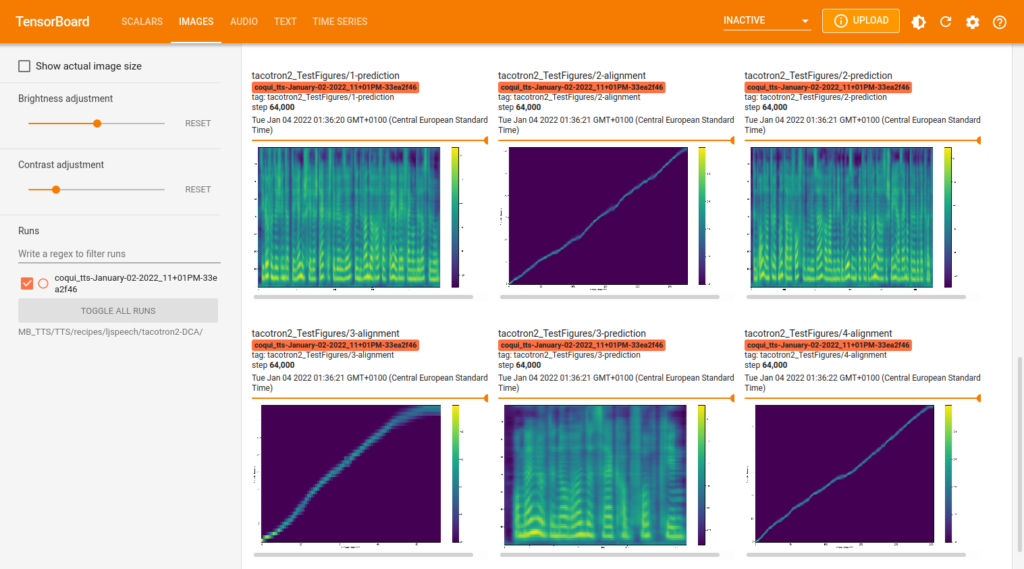
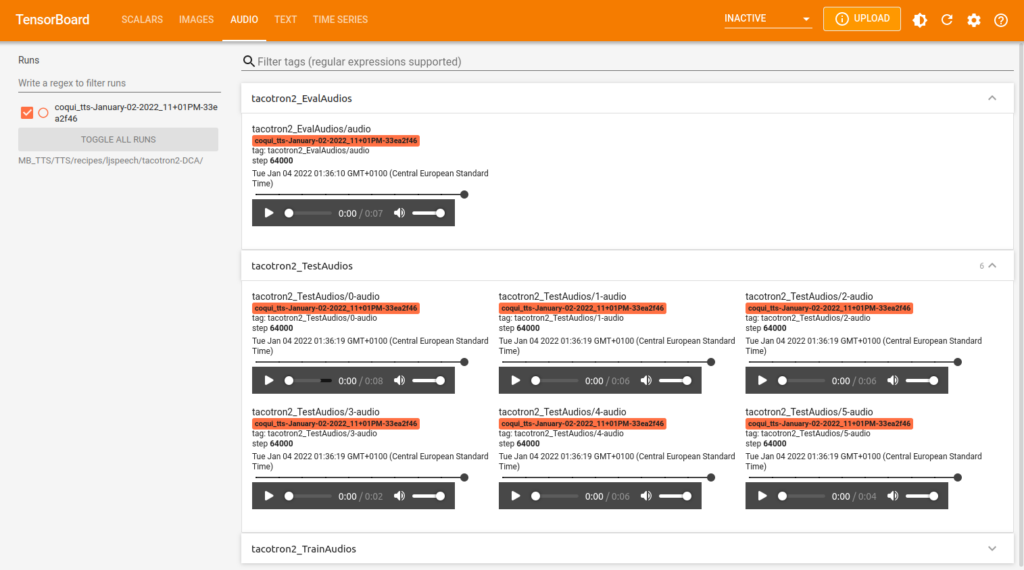
On peut également consulter les paramètres de configuration en vigueur pour l’entraînement dans le menu “Text”.
Google, le créateur des outils TensorFlow et TensorBoard, offre une plateforme gratuite de partage des résultats d’expériences d’apprentissage profond, sous le nom de TensorBoard.dev. Malheureusement cette plateforme ne permet pas encore le partage des spectrogrammes et des données audio. J’ai toutefois installé mon livre de bord relatif au modèle Tacotron2-DCA sur cette plateforme, ce qui permet à chacun d’analyser l’évolution des scalaires afférents en temps réel.
La prochaine image donne un aperçu sur cet outil TensorBoard.dev.
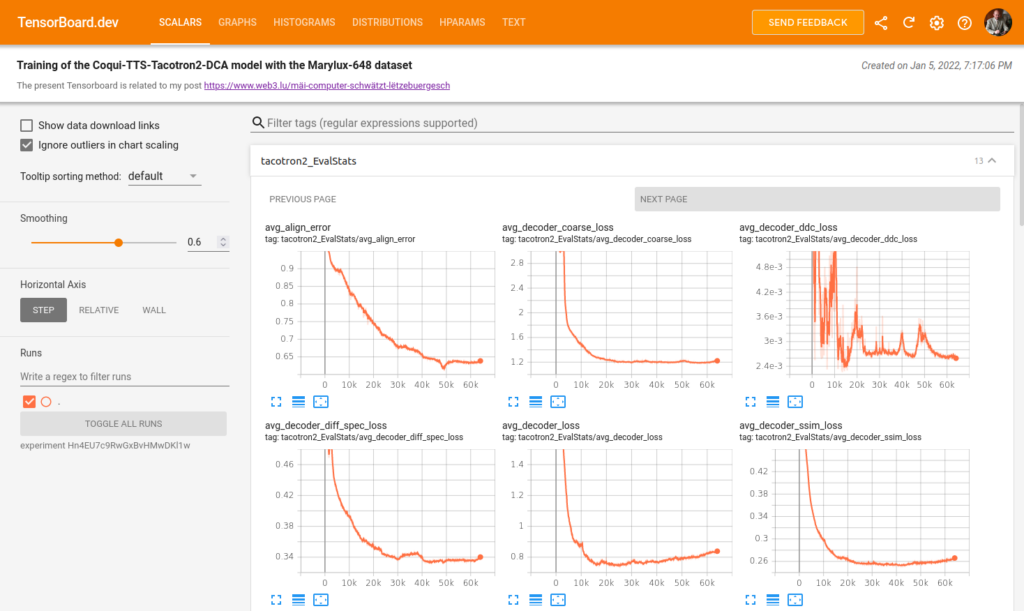
Sans vouloir entrer dans les détails, l’exploration du livre de bord montre que l’entraînement dun modèle TTS Tacotron2-DCA avec la base de données Marylux-648 est entré dans une phase de sur-apprentissage (overfitting), ce qui ne permet pas de synthétiser correctement des mots ou phrases non vus lors de l’apprentissage. Il faut se rendre à l’évidence que la taille de la base de données Marylux-648 est insuffisante pour entraîner un modèle TTS Tacotron2-DCA.
Il est vrai que Tacotron est connu pour être gourmand en données.
Je vais présenter les résultats obtenus avec d’autres modèles TTS lors de prochaines contributions sur mon présent site web. Mais pour ne pas laisser le lecteur intéressé sur sa faim jusque-là, je vais rapidement introduire le dernier né des familles TTS neuronaux. Il s’appelle VITS.
Marylux-648 VITS
VITS est l’abréviation pour Conditional Variational Autoencoder with Adversarial Learning for End-to-End Text-to-Speech. La prochaine figure montre les signaux temporels des six phrases de la fable “Nordwand”, synthétisées avec le modèle Coqui-TTS VITS. L’allure donne confiance, et l’audition qui suit confirme que la qualité de la synthèse dépasse largement celle du modèle Tacotron2-DCA.
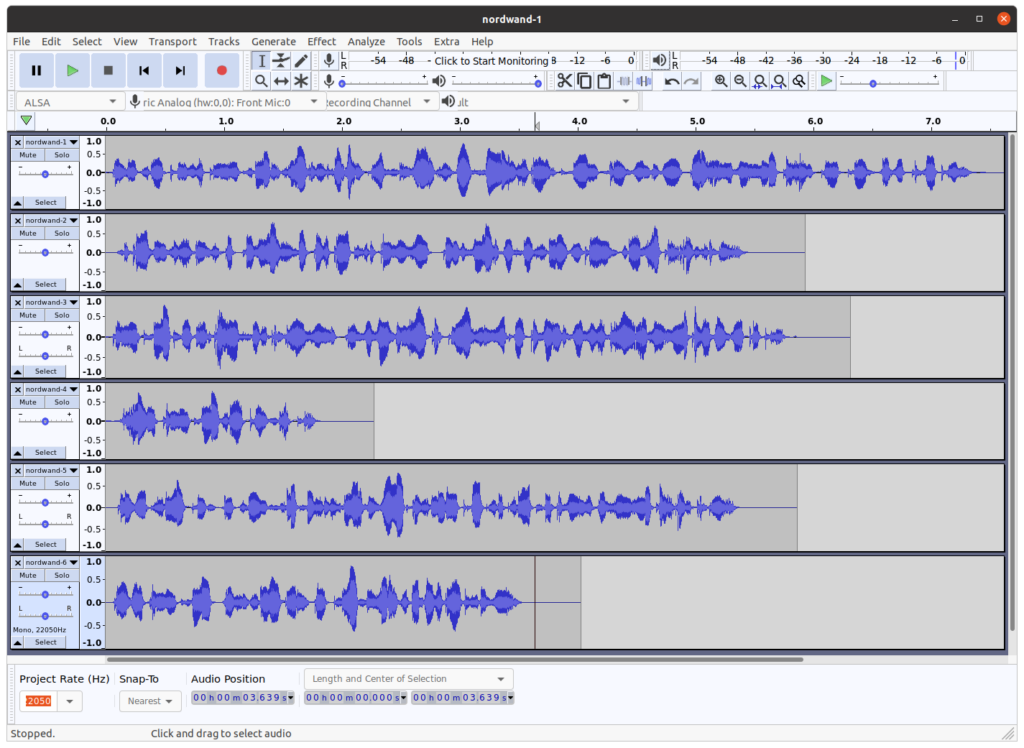
Les deux figures qui suivent montrent un aperçu de l’évolution des métriques lors de l’entraînement de la base de données Marylux-648 avec le modèle TTS VITS. Les graphiques peuvent être visualisés en détail sur TensorBoard.dev.
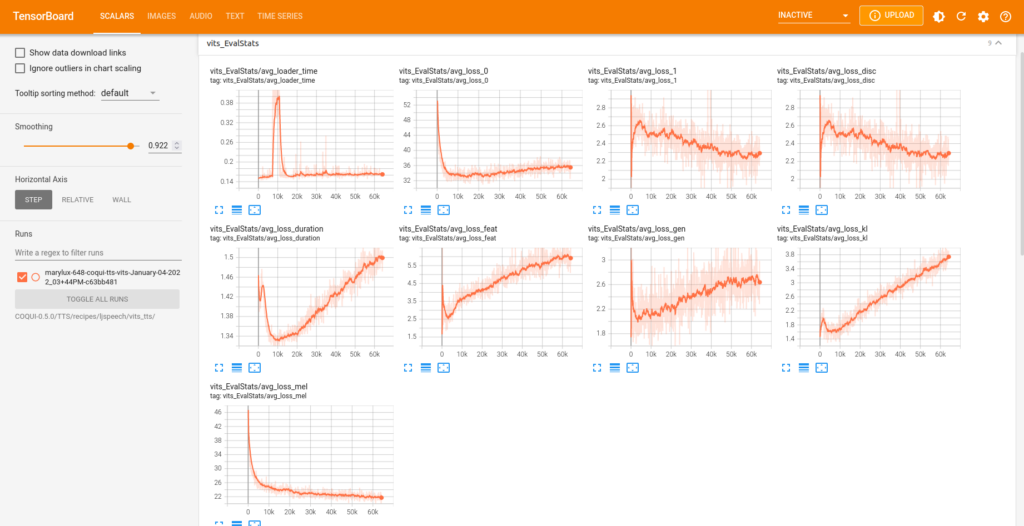
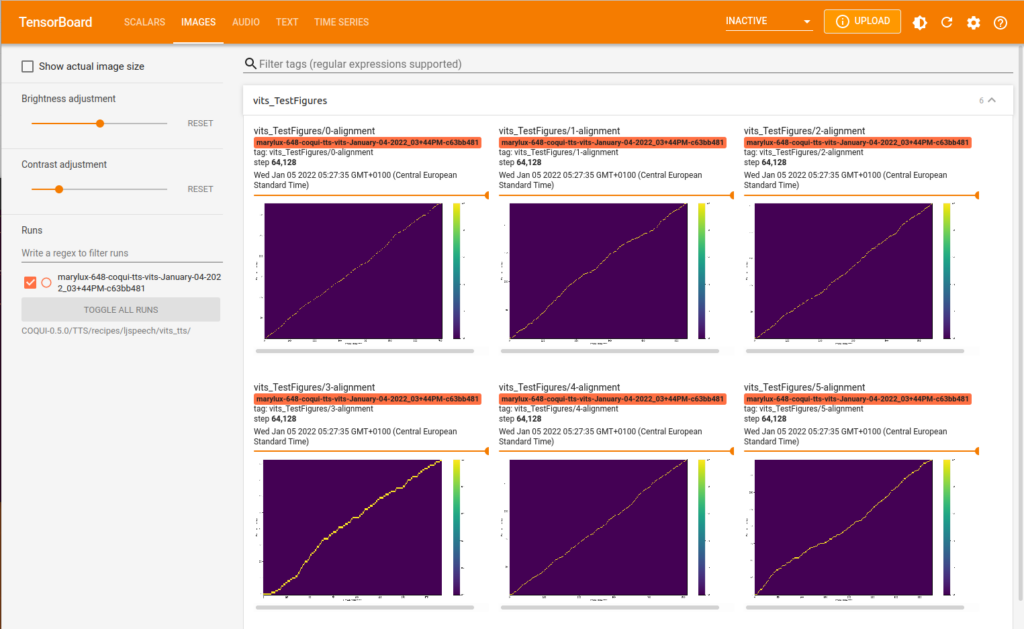
Mot de la fin
L’apprentissage profond de machines est une technique passionnante. Le comportement des réseaux neuronaux à la base des architectures de systèmes de synthèse de la parole me rappellent parfois les réactions de mes cinq petits-enfants lorsqu’ils faisaient de nouvelles découvertes ou lors d’un nouvel apprentissage. J’ai également constaté que des anciennes techniques réapparaissent dans des nouveaux systèmes. Les spectrogrammes utilisés dans les modèles neuronaux TTS ont déjà été utilisés dans la machine Pattern Playback, développé par Franklin S. Cooper à la fin des années 1940.
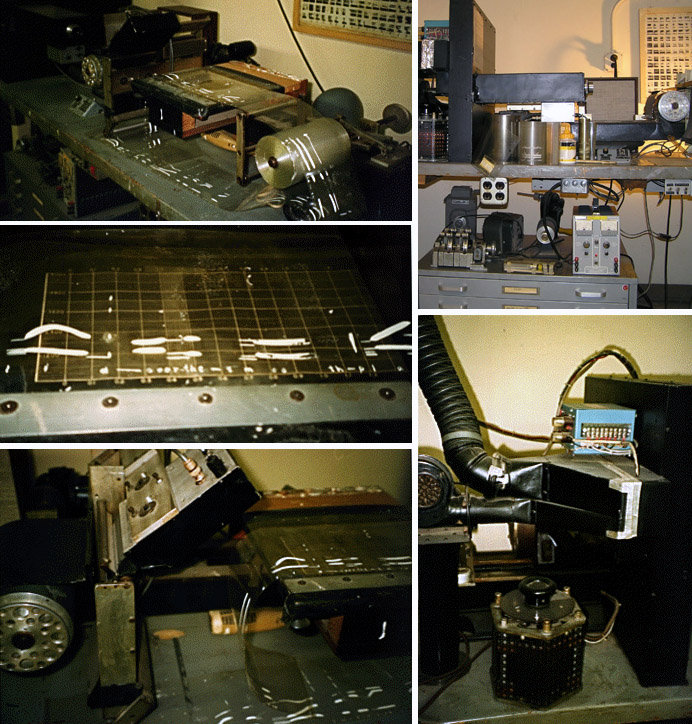
Liens
- The Best of two Breeds, mbarnig, 17.6.2021
- Synthèse de la parole : Histoire de la synthèse vocale mécanique, électrique, électronique et informatique, Marco Barnig, 2020
- Luxemburgistik 2.0, Lëtzebuerger Land , 2.8.2019
- Language : fr, de, en, lb, eo, mbarnig, 20.9.2014